Cato CTRL Threat Research: Advanced Behavioral Analysis of IoT and OT Devices for IoC Collection
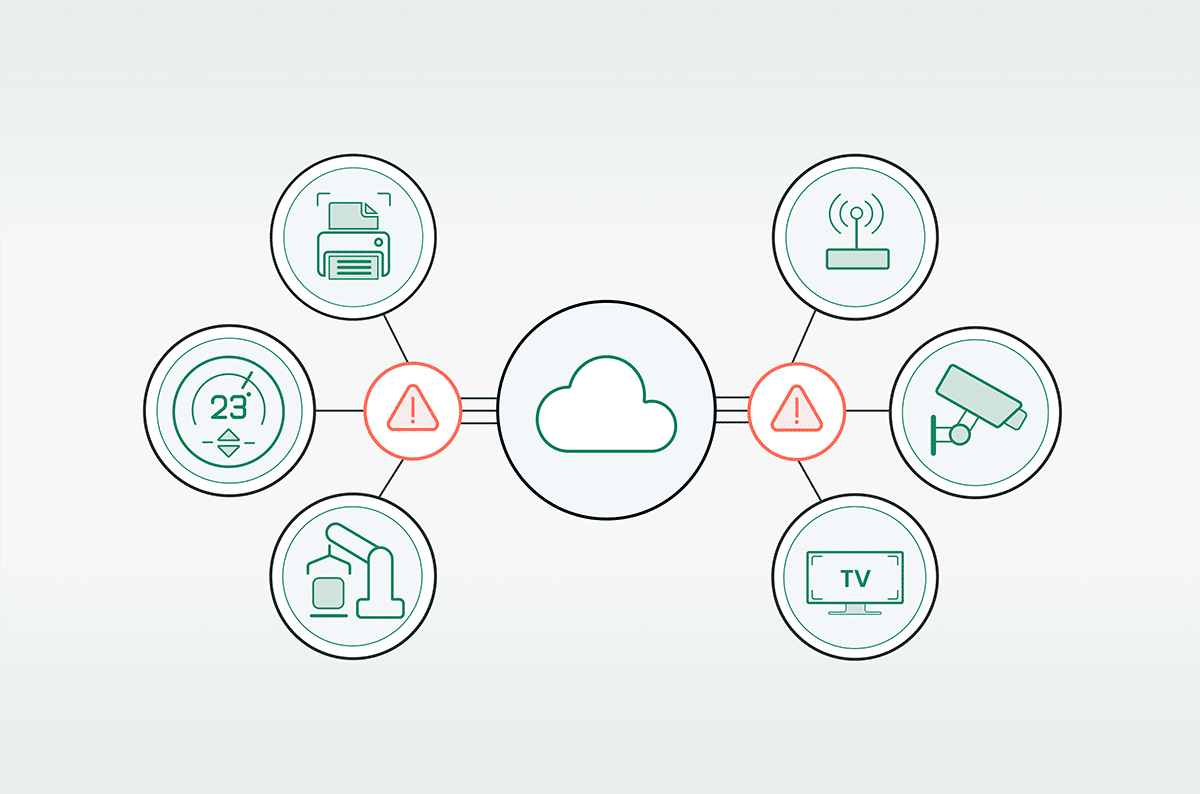
Table of Contents
Listen to post:
Getting your Trinity Audio player ready...
|
Executive Summary
In today’s hyperconnected industrial landscape, IoT and OT devices have become integral to modern operations, yet their security posture often remains inadequately monitored.
Cato CTRL is revealing a new and innovative approach to IoT/OT threat detection by leveraging advanced behavioral analysis of IoT/OT devices to generate high-confidence Indicators of Compromise (IoCs). We’ve developed a detection methodology for the Cato SASE Cloud Platform capable of identifying suspicious activities without relying on pre-existing threat intelligence, allowing the detection of novel threats and previously unknown attack patterns in IoT/OT environments.
This implementation successfully identifies dozens of new IoCs weekly, which are subsequently validated against established threat intelligence sources and automatically integrated into Cato IPS. Perhaps most notably, the methodology we’ve developed is device-agnostic and highly scalable, making it applicable across various device types and organizational environments.
This blog details our approach, findings, and implications for the broader security community, offering insights into how organizations can enhance their security posture through advanced behavioral analysis of IoT/OT devices.
Q2 2024 IoT/OT security that works as hard as your devices | Get the White PaperTechnical Overview
Our detection methodology employs a comprehensive process that evolves from initial traffic monitoring to active threat prevention. Each stage builds upon the insights gained from the previous ones, creating a robust security framework.
Figure 1. Illustration of the complete workflow, which demonstrates how we progress from device discovery through behavioral analysis to eventual threat mitigation
Let’s examine each stage in detail to understand how they work together to provide effective protection for IoT and OT devices against emerging threats.
The foundation of our detection methodology rests on a sophisticated device discovery and classification system, powered by purpose-built artificial intelligence (AI) and machine learning (ML) capabilities. This system provides detailed visibility into IoT and OT device characteristics, including type, manufacturer, and version information—creating a framework for precise behavioral analysis in production environments.
The system automatically converts validated threat indicators from IoT and OT communications into Cato IPS, which is deployed across the security infrastructure. This protects all Cato-connected edges—sites, remote users, and cloud resources. The automation eliminates the traditional lag between threat detection and mitigation, a critical advantage in protecting IoT and OT environments where vulnerabilities can be rapidly exploited.
Our behavioral baseline establishment employs a dual-track approach, combining individual IoT and OT device analysis over several weeks with peer group comparison across similar devices within the organization. This comprehensive method ensures accurate baseline creation by minimizing the impact of temporary anomalies, while leveraging statistical patterns from multiple peer devices for enhanced reliability.
The framework analyzes multiple communication attributes to detect anomalous behavior, encompassing both basic network parameters and complex interaction patterns. By evaluating connections based on destination IP addresses, applications, autonomous system numbers (ASN), geographical locations, and traffic directionality, the system compares individual IoT and OT device activities against peer group baselines using statistical analysis for precise anomaly detection.
Figure 2 below presents our key detection features and their associated risk scores. Features with “High” risk scores can independently trigger alerts for suspicious communications, while features with “Medium” or “Low” risk scores must occur in combination with other features to generate an alert.
Figure 2. Key detection features and their associated risk scores
When the system identifies potentially suspicious communications, it initiates an automatic multi-stage validation process. This process cross-references findings against established threat intelligence platforms, including IPQualityScore, VirusTotal, AbuseIPDB and Webroot, ensuring high confidence in the identified indicators before they’re propagated to prevention systems. This automated validation workflow significantly reduces the operational overhead typically associated with threat intelligence generation.
Results and Impact
To demonstrate our methodology’s effectiveness, let’s examine a real-world use case from a US-based insurance provider involving VoIP devices.
Our system first established behavioral baselines for Yealink VoIP devices by analyzing their typical network patterns, including common IP destinations, regular applications, geographic locations of remote servers, and expected inbound \ outbound traffic patterns. During monitoring, we identified a specific Yealink VoIP device that exhibited multiple concerning deviations from normal behavior. This device initiated communications with servers in previously unseen countries, connected to multiple IP addresses that its peer devices had never contacted, and interacted with a new Autonomous System Number (ASN). These multiple anomalies from the same device, when analyzed collectively, indicated potentially suspicious behavior. The suspicious IP addresses were automatically extracted and cross-referenced with threat intelligence databases, enabling rapid response through our protection mechanisms in Cato IPS.
Figure 3. Detection of anomalous Yealink VoIP behavior and IoC extraction
Conclusion
Behavioral analysis represents a fundamental advancement in IoT and OT security. By moving beyond traditional signature-based detection methods, we’ve developed a detection methodology that adapts to the evolving threat landscape while maintaining the reliability required by enterprise environments.
Our detection methodology automatically identifies and responds to new threats with consistently high accuracy, effectively addressing the growing challenge of securing increasingly complex device ecosystems.